In the realm of Artificial Intelligence (AI) and Machine Learning (ML), data is the lifeblood that fuels innovation. The process of data preparation for AI, often underestimated, is a critical stepping stone towards achieving accurate and actionable insights.
This article explores the intricacies of data preparation, shedding light on its importance, challenges, and best practices.
The Essence of Data Preparation for AI
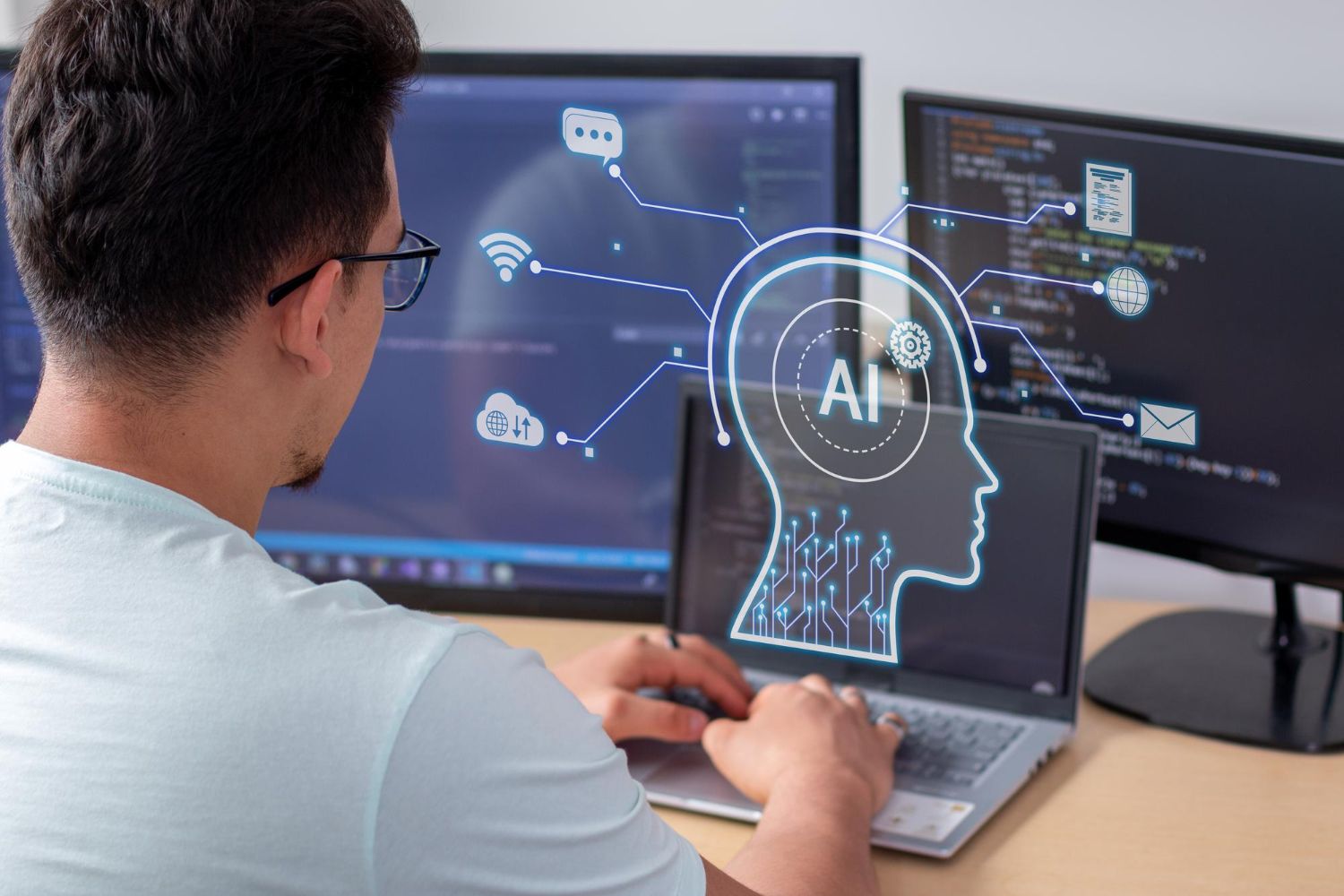
Data preparation for AI involves the meticulous process of collecting, cleaning, transforming, and organizing raw data into a format suitable for machine learning algorithms. This process is the bedrock upon which successful AI models are built.
1. Data Collection and Sourcing
Gathering relevant and representative data from diverse sources is the initial phase of data preparation. It’s essential to ensure data quality and diversity to avoid bias.
2. Data Cleaning and Preprocessing
Data often comes with inconsistencies, missing values, and noise. Data cleaning involves rectifying these issues to ensure accurate and reliable insights.
3. Feature Engineering
Feature engineering transforms raw data into features that machine learning algorithms can understand. This step enhances the predictive power of AI models.
4. Data Transformation and Normalization
Data transformation includes scaling and normalizing features to bring them within a consistent range, ensuring fair treatment for different variables.
5. Handling Categorical Data
Categorical data requires encoding to make it suitable for machine learning algorithms. Techniques like one-hot encoding and label encoding are used.
6. Dealing with Imbalanced Data
Imbalanced datasets can skew AI models’ performance. Techniques like oversampling, undersampling, and Synthetic Minority Over-sampling Technique (SMOTE) address this challenge.
The Significance of Data Preparation
Data preparation for AI serves as the foundation for successful model building:
1. Improved Model Accuracy
Clean, well-prepared data leads to more accurate and reliable AI models, enhancing their predictive power.
2. Enhanced Generalization
Quality data enables models to generalize well to new, unseen data, reducing overfitting.
3. Efficient Training
Well-prepared data accelerates model training, reducing the time and resources required.
4. Optimal Resource Utilization
Clean data ensures that computational resources are focused on meaningful patterns rather than noise.
Data Preparation Challenges and Strategies
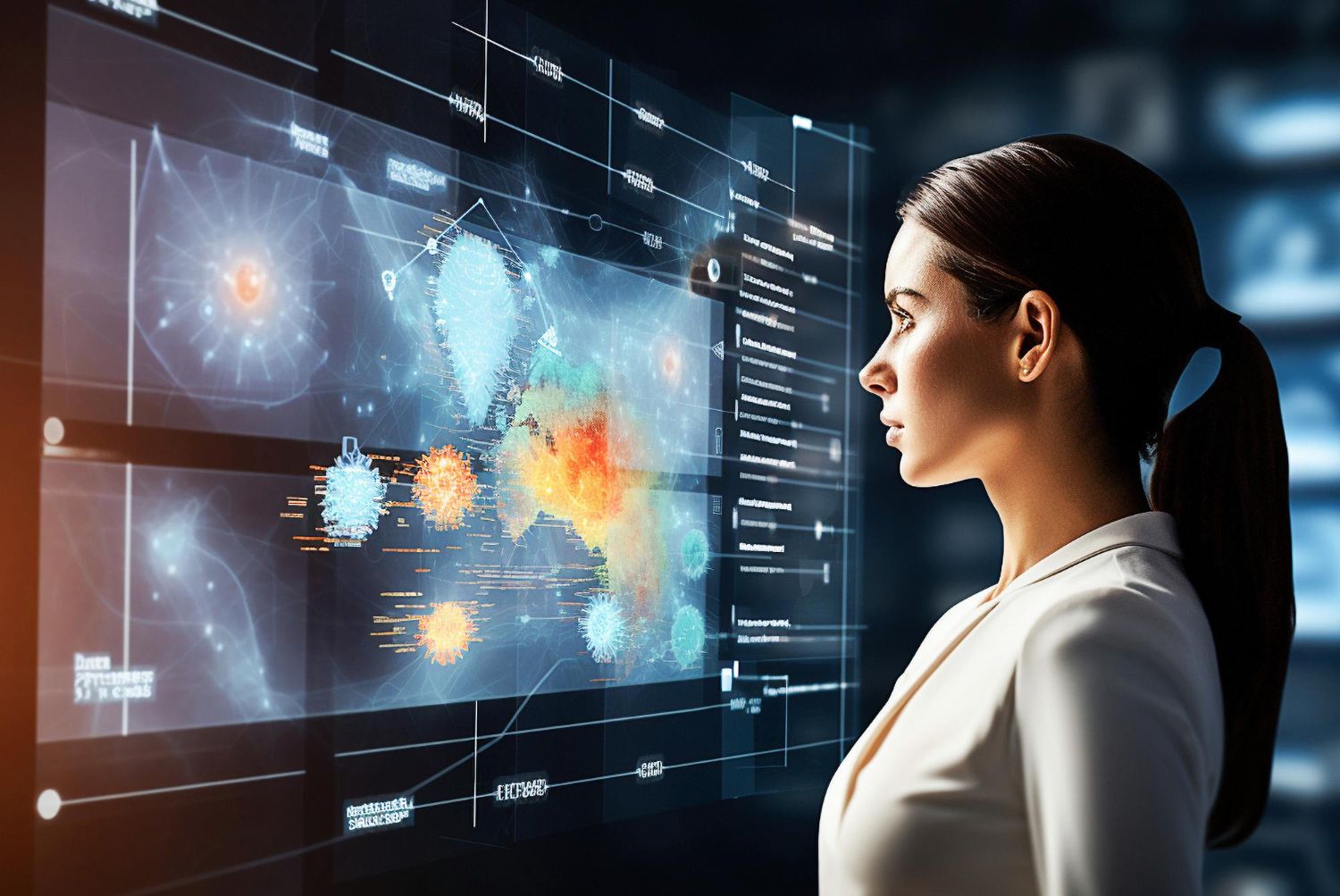
Data preparation isn’t without its challenges:
1. Data Quality
Ensuring data accuracy, consistency, and completeness is crucial. Data profiling tools can help identify data quality issues.
2. Scalability
Scalable data preparation techniques are required to handle large and complex datasets.
3. Automation
Automating data preparation processes can reduce manual effort and streamline the workflow.
Best Practices for Effective Data Preparation
Adhering to best practices is essential for successful data preparation:
1. Understand the Data
Thoroughly understand the dataset’s structure, relationships, and potential challenges.
2. Implement Version Control
Maintain different versions of the prepared dataset for reproducibility and traceability.
3. Data Validation
Validate the prepared dataset using cross-validation techniques to ensure its accuracy.
4. Continuous Monitoring
Regularly monitor data quality to detect anomalies or shifts that may affect model performance.
The Future of Data Preparation
As AI continues to evolve, data preparation will also undergo advancements:
- Automated Feature Selection: AI-driven feature selection algorithms will streamline the selection of relevant features.
- Self-Service Data Preparation Tools: Non-technical users will benefit from self-service tools that simplify data preparation.
Embracing the Data Preparation Journey
Data preparation for AI is not a one-time task; it’s an ongoing journey that requires dedication and expertise. Organizations that prioritize data preparation set the stage for AI success:
1. Cultivating Data Literacy
Nurturing a data-literate culture ensures that everyone understands the significance of accurate data.
2. Investing in Data Professionals
Data professionals play a pivotal role in ensuring data quality, integrity, and compliance.
3. Collaboration
Collaboration between data scientists, engineers, and domain experts enhances data preparation effectiveness.
In conclusion, data preparation for AI is the unsung hero behind AI’s success. The diligence invested in collecting, cleaning, and transforming data lays the groundwork for insightful AI models. By recognizing the importance of data preparation, organizations can unlock the full potential of their AI initiatives, ushering in a future where data-driven decisions are more informed, reliable, and impactful.