Laboratories are expected to meet high precision, speed, and efficiency in data processing and documentation. Laboratory data reconciliation tracks sample information and ensures regulatory standards are met. The manual methods of data reconciliation work are also practiced but they are prone to errors, are time-consuming, and may easily become a bottleneck in your workflow.
Automating Lab Data Reconciliation
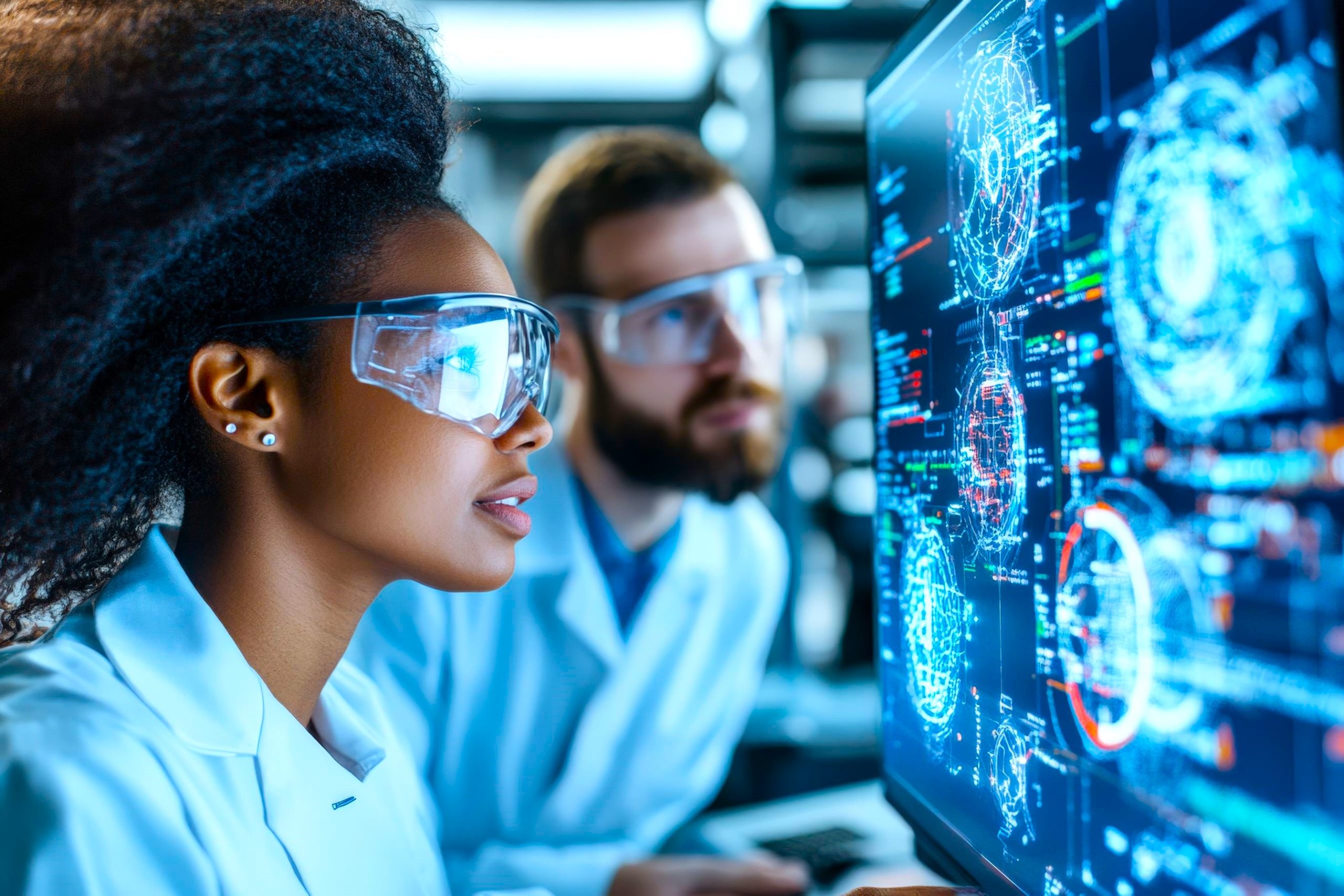
Automation in laboratory data reduces the possibility of human error and increases the speed of data validation. Laboratories can leverage modern tools to transform their operations without compromising data integrity while unleashing significant efficiency gains.
Here are some key steps labs can take to successfully automate their data reconciliation processes and achieve better outcomes.
Assess Current Data Reconciliation Processes
The first step in reconciliation is assessing the current state of your reconciliation process. If you review the existing workflows then it will help you look for inefficiencies or problematic points. Are there specific steps in the process where errors tend to frequently occur? Are there bottlenecks that impede the reconciliation of critical data?
Currently, over 70% of trial data is in non-CRF form, meaning you may end up with dirt data and no audit trail. Therefore, look into how to automate lab data reconciliation through a reputable data exchange program that helps with transfer, standardization, and compliance. For instance, if your current process uses email chains to validate specifications, then you’ll need a platform that allows you to create, edit, and verify specs collaboratively in one central place.
Thorough assessment enables labs to establish the foundation upon which comparison will be based. The idea is to have today’s performance metrics documented so that improvements due to automation can be measured over a period. This baseline data analysis is important to ensure the automation initiative is customized for the needs of the lab and lays a sound foundation for success.
Define Goals and Success Metrics
Clear goals are essential for any successful automation project. When automating lab data reconciliation, labs must first define what they hope to achieve. Are they primarily looking to reduce errors? Speed up data processing? Improve compliance? Specific, measurable objectives keep automation efforts focused and aligned with the overall operational goals.Â
Success metrics also provide a yardstick upon which the effectiveness of the automation process will be gauged. Helpful ones include error rates, time to reconcile data, and the number of manual interventions required post-automation. Compliance metrics will also need consideration by laboratories to ensure their automated processes meet applicable regulatory standards.
Defining goals and metrics at the outset helps drive decision-making throughout the automation process. It ensures that every decision—whether it’s selecting tools or training staff—is aligned with the desired outcomes.
Select the Right Technology
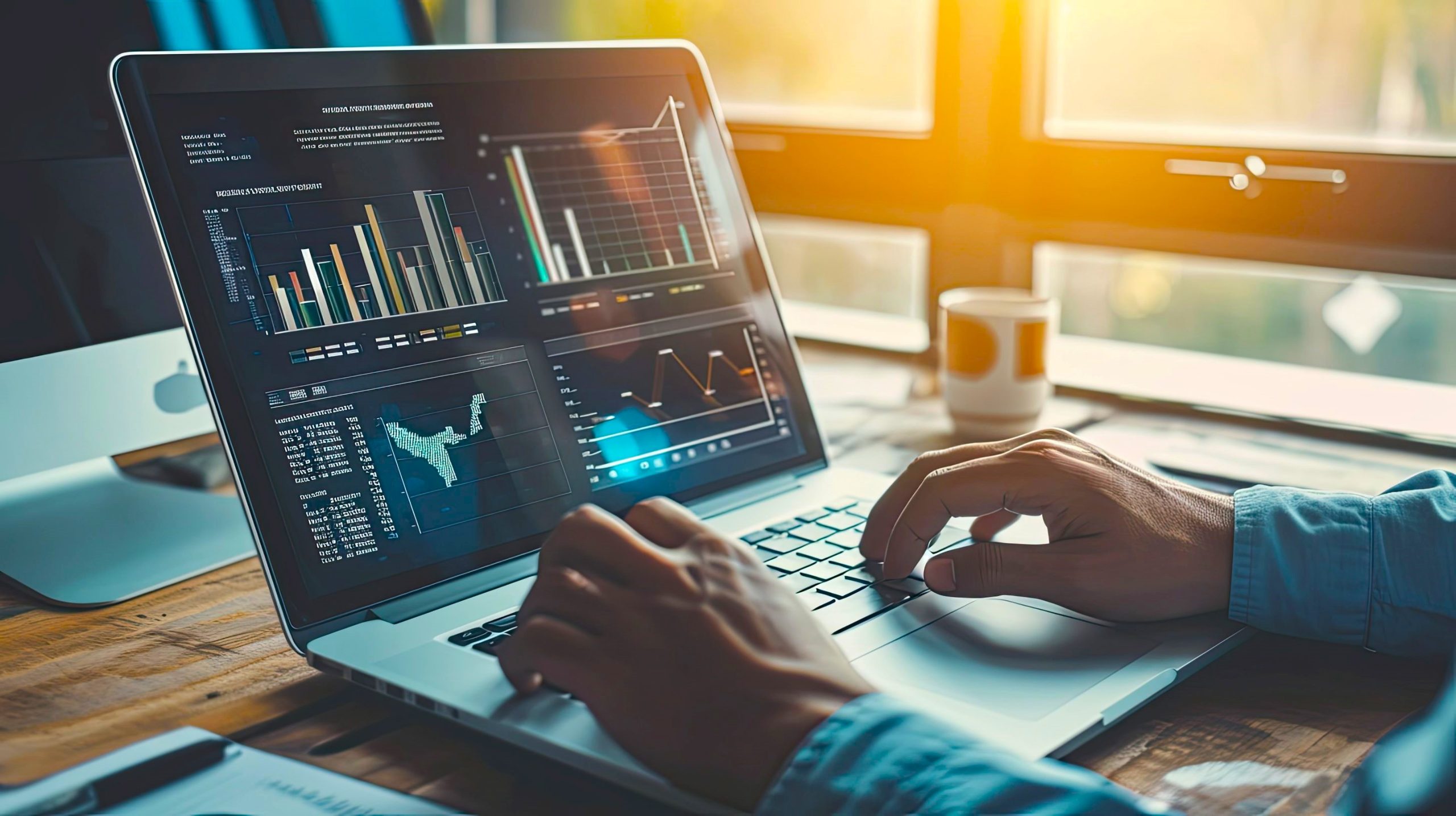
Choosing the right technology is perhaps the most critical step in lab data reconciliation automation. With a plethora of solutions at their fingertips, laboratories need to make careful choices. Key features to look for include data integration capabilities, error detection and correction functionalities, and robust reporting tools.
Compatibility with the existing lab system is another critical consideration. Many labs already use systems such as LIMS or ERP software. The selected reconciliation tool should be able to seamlessly integrate with these programs to avoid creating additional silos or inefficiencies.
Finally, laboratories should consider the technology choice from a long-term perspective. This involves only selecting tools that will offer ongoing support and updates, as well as adapting to upcoming tech upgrades.
Ensure Data Consistency and Quality
Most automation initiatives depend on two prime factors: data consistency and quality.
Low-quality data, such as incomplete, inconsistent, or outdated records, can diminish the efficiency of even the most advanced reconciliation tool. Laboratories should invest time in data acquisition, cleaning, and standardization before actually implementing automation.
Moreover, laboratories constantly create new data or update previous ones, which means that continuous monitoring and validation of the data are required if it is to remain accurate and consistent.
Train Staff and Implement Change Management
Automating lab data reconciliation isn’t just about implementing new tools. It’s also about ensuring that the people using them are fully prepared and onboard.
Training staff is a crucial step in the automation process. Lab personnel need to understand not only how to use the new system but also why the changes are being made.
Change management is equally important. Resistance to change is a common challenge when introducing new technologies. To overcome this, labs should involve staff in the automation process from the beginning, seeking their input and addressing their concerns. Clear communication about the benefits of automation—such as reduced workloads and improved data accuracy—can also help foster buy-in and enthusiasm among team members.
Conclusion
Automating lab data reconciliation is a transformative step that can significantly improve efficiency, accuracy, and compliance in laboratory operations. Key steps such as assessing current processes, defining goals, and selecting the right tools are crucial for data validation success. Embracing automation isn’t just about improving workflows today; it’s also about preparing for the future of laboratory science.